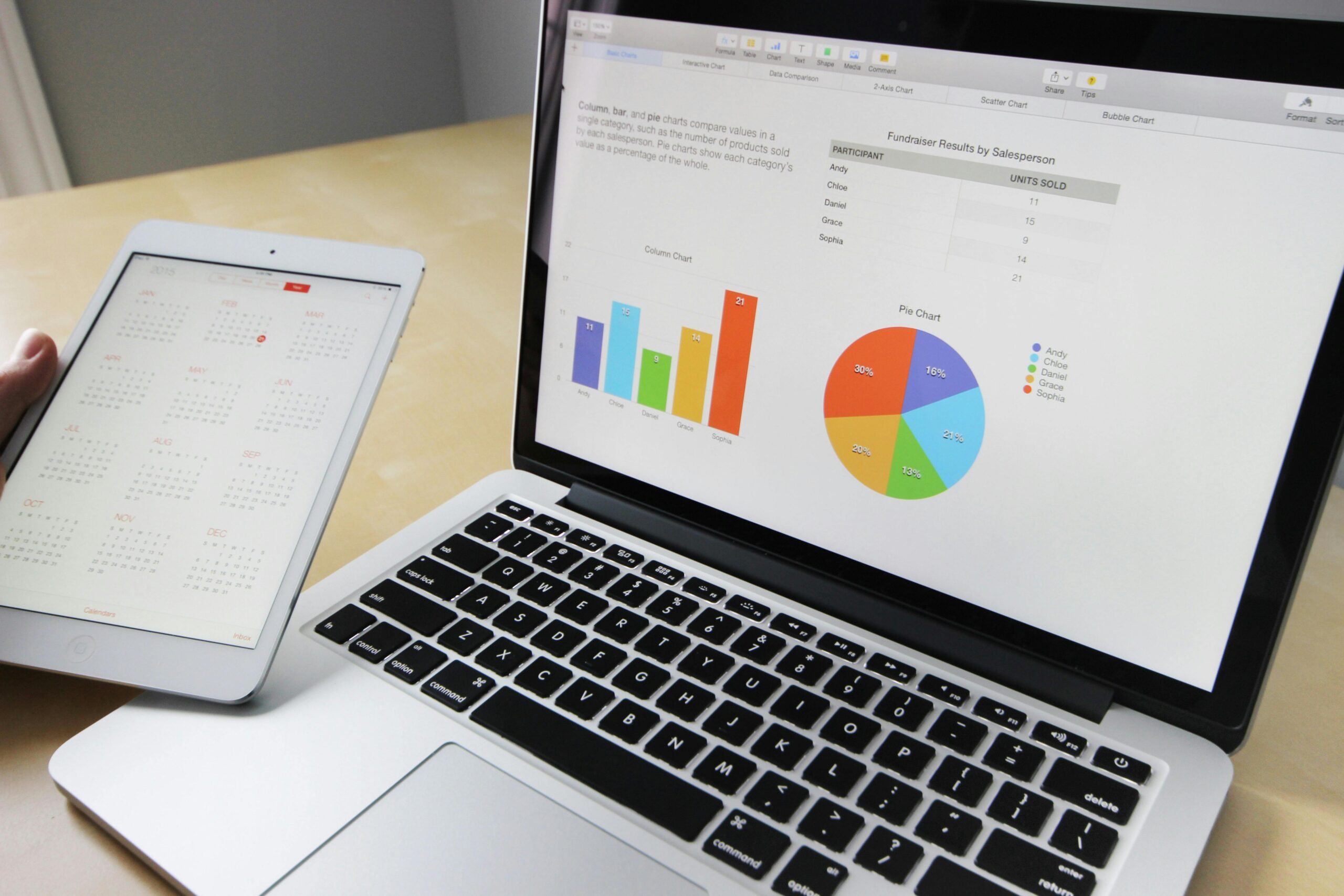
Introduction:
This course equips individuals and professionals with the necessary skills to navigate the exciting world of data analysis. Through a blend of engaging lectures, interactive exercises, hands-on projects, and real-world case studies, you’ll gain the knowledge and practical experience needed to transform raw data into actionable insights. Whether you’re a beginner with no prior coding experience or a seasoned professional seeking to refine your skills, this course caters to diverse learning needs
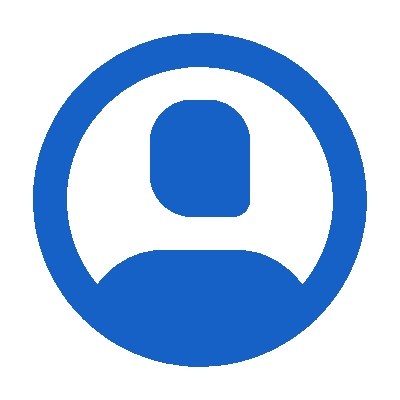
Target Audience:
- Anyone interested in using data to drive organisational growth
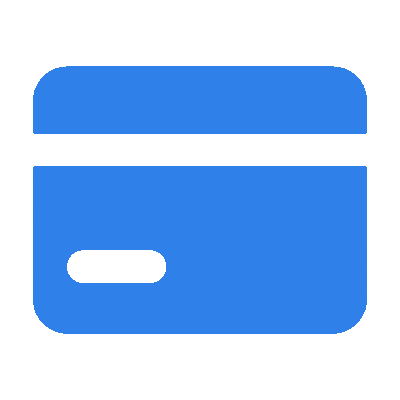
Price:
₦500,000
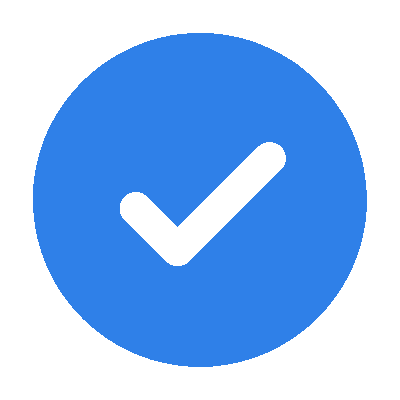
Course Benefits:
- Gain a comprehensive understanding of data analysis principles, methodologies, and tools.
- Develop practical skills in data cleaning, manipulation, and analysis using a chosen programming language.
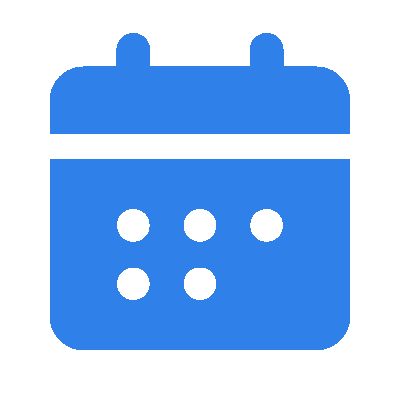
Course Duration:
16 Weeks program
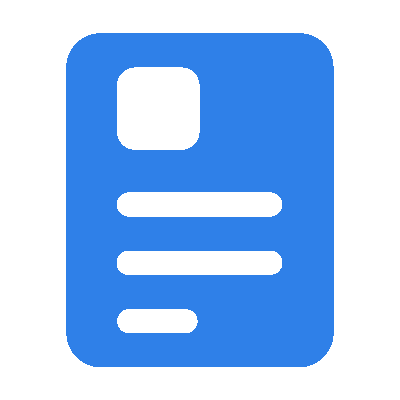
Course Format:
- Engaging Lectures: Clear explanations of data analysis concepts, methodologies, and tools.
- Interactive Exercises and Quizzes: Applying data analysis techniques to real-world datasets.
- Hands-on Projects: Building practical skills through individual and collaborative data analysis projects.
- Real-World Case Studies: Exploring successful applications of data analysis across various industries.
- Discussion Forums and Q&A Sessions: Collaborative learning and addressing student questions.
- Career/Internship: CV development, interview prep and work experience
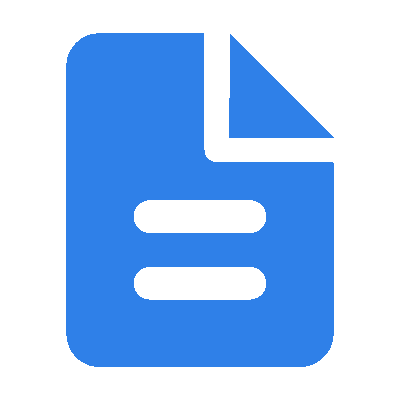
Course Outline:
Module 1: Data Foundations (Understanding Your Data)
- Define data, its various types (structured, unstructured, semi-structured), and their importance.
- Explore the Data Lifecycle (acquisition, storage, processing, analysis, visualization).
- Discuss data quality concepts (accuracy, completeness, consistency) and data cleaning techniques.
- Introduce essential data analysis tools and technologies (Excel, Power Query, SQL, Python, etc.).
Activities:
- Interactive exercises on data classification and lifecycle stages.
- Hands-on labs practicing data cleaning methods in a common tool (Excel/Python).
- Group discussions on data quality challenges and best practices.
Module 2: Data Sources: Where Does Your Information Come From?
- Define data, its various types (structured, unstructured, semi-structured), and their importance.
- Explore the Data Lifecycle (acquisition, storage, processing, analysis, visualization).
- Discuss data quality concepts (accuracy, completeness, consistency) and data cleaning techniques.
- Introduce essential data analysis tools and technologies (Excel, Power Query, SQL, Python, etc.).
Activities:
- Interactive exercises on data classification and lifecycle stages.
- Hands-on labs practicing data cleaning methods in a common tool (Excel/Python).
- Group discussions on data quality challenges and best practices.
Module 3: Data Exploration & Analysis (Uncovering Insights)
- Introduce Exploratory Data Analysis (EDA) techniques for understanding data characteristics (descriptive statistics, visualizations).
- Cover data wrangling methods for preparing data for analysis (data transformation, feature engineering).
- Discuss common data analysis techniques (hypothesis testing, correlation analysis).
Activities:
- interactive workshops on calculating descriptive statistics using a chosen tool (Excel/Power Query/Python).
- Hands-on labs practicing data wrangling techniques and data analysis methods.
- Group projects conducting basic EDA and analysis on a provided dataset.
Module 4: Data Visualization (Communicating Your Findings - Part 1)
- Explain the importance of data visualization in communicating insights effectively.
- Introduce visual perception principles and best practices for creating clear and compelling visualizations.
- Cover common data visualization techniques (bar charts, histograms, scatter plots, line charts) and their use cases.
Activities:
- Interactive exercises on applying visual perception principles to data visualizations.
- Hands-on workshops on creating various data visualizations using a chosen tool (Excel/Tableau/Power BI).
- Group discussions on selecting the right visualizations for different data types and analysis goals.
Module 5: Data Visualization (Communicating Your Findings - Part 2)
- Introduce advanced data visualization techniques (heatmaps, box plots, pie charts, network graphs) and their applications using Power BI.
- Discuss interactive dashboards and storytelling techniques for presenting data insights.
- Explore data visualization best practices for accessibility and ethical considerations.
Activities:
- Hands-on labs on creating advanced data visualizations using a chosen tool.
- Group projects on designing interactive dashboards to communicate data-driven stories.
- Case studies analyzing effective and ineffective data visualizations from real-world examples.
Module 6: Data Storytelling (The Power of Narrative)
- Explain the art of data storytelling: crafting a narrative using data to engage the audience and influence decisions.
- Discuss the key elements of a compelling data story (context, evidence, insights, recommendations).
- Cover effective communication techniques for presenting data insights clearly and concisely.
Activities:
- Interactive exercises on identifying the elements of a strong data story.
- Group projects on developing data stories from provided datasets.
- Peer-review sessions on refining data storytelling techniques.
Module 7: Data for Decision Making (The Power of Insights)
- Explain how data analysis helps organizations make informed and data-driven decisions.
- Discuss real-world examples of data impacting business outcomes (marketing campaigns, product development, customer service).
- Introduce key performance indicators (KPIs) and their role in measuring data-driven success.
- Explore potential challenges and biases in data analysis and how to mitigate them.
Activities:
- Case studies analyzing how companies have used data to achieve business goals.
- Group projects on identifying potential data-driven solutions to a business challenge.
- Interactive exercises on identifying potential biases in data and decision-making.
Module 8: Harnessing Automation for Business Success
- Explain the benefits of automation in data analysis tasks (data collection, cleaning, reporting).
- Discuss different data automation tools and technologies (e.g., ETL/ELT tools, Python scripts).
- Explore best practices for implementing data automation solutions within organizations.
- Identify potential challenges and limitations of data automation.
Activities:
- Case studies analyzing how companies have used automation to improve data analysis efficiency.
- Hands-on tutorials on basic data automation techniques using a chosen tool.
- Group discussions on identifying tasks for automation within a specific business scenario.
Module 9: Understanding the Customer Data Journey & Decision Methodology
- Explain the concept of the customer data journey and its touchpoints.
- Discuss various methods for collecting customer data at different stages of the journey.
- Analyze customer decision-making processes and how data can be used to influence them.
- Explore strategies for personalization and targeted marketing based on customer data insights.
Activities:
- Interactive exercises on mapping the customer data journey for a specific product or service.
- Group projects on developing customer personas based on data analysis.
- Case studies analyzing how companies have used data to personalize customer experiences.
Module 10: Building a Robust Data Culture
- Define a data-driven culture and its core characteristics (data literacy, collaboration, open communication).
- Discuss the importance of fostering a culture of data-driven decision-making across the organization.
- Explore strategies for promoting data literacy and encouraging data exploration among employees.
- Identify potential challenges in building a data culture and how to overcome them.
Activties:
- Interactive exercises on identifying key principles of a data-driven culture.
- Group discussions on developing strategies for promoting data literacy within an organization.
- Role-playing exercises on communicating data insights to teams with varying levels of data expertise.